This report is available exclusively to subscribers of Inman Intel, a data and research arm of Inman offering deep insights and market intelligence on the business of residential real estate and proptech. Subscribe today.
A picture might be worth a thousand words. But who has the time to translate?
Barcelona-based Restb.ai, which deploys artificial intelligence to extract data from still and motion images, is attempting to answer that question by using listing photos to tell deeper stories about homes — and the markets they’re in.
Insights derived from a picture, according to the company, can explain why a listing stayed on the market 12 days longer than its comparables. The ability to compare images of rooms in competing homes can also help a buyer narrow the field of listings in much less time than a series of actual driving tours.
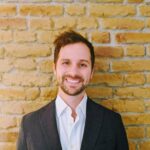
Nathan Brannen, Restb.ai CPO
So as OpenAI’s ChatGPT continues to draw agents for its language skills, Restb.ai’s computer vision technology is on the precipice of impacting real change in the industry, Chief Product Officer Nathan Brannen told Intel.
“There are about a million photos uploaded everyday in the U.S., just in MLSs,” he told Intel. “Someone may buy a home without seeing it, but no one buys a home without seeing a photo of it. They contain so much information.”
In a conversation edited for length and clarity, Brannen revealed why Restb.ai chose real estate as its focus, what limitations computer vision has, the promise it holds for sales and the remarkably bad photos agents use to market homes.
Intel: How did you know computer vision would have so many valuable applications for real estate?
Brannen: Speaking with the founders when I joined the company, I asked this, too. From their perspective, they had trouble finding a house, and while people complain about certain things in the U.S., I can promise you they can be worse [in Barcelona].
They did look at other spaces, such as automotive and the classified space, they really looked at what space has the most number of images that have the largest impact on decisions.
If you look at e-commerce, there are lot of photos, but it’s not as impactful as buying a home. There are about a million photos uploaded everyday in the U.S. just in MLSs, and then if you look at photos uploaded in property insurance and appraisals, that number multiplies. You’re making the biggest decision of your life on these photos.
Someone may buy a home without seeing it, but no one buys a home without seeing a photo of it. They contain so much information.
How did you manage to get MLSs on board? They’re traditionally a very hard sell.
One of the first companies we had on board was Idealista, the Zillow of Spain. So, we already had one of the largest clients we could have in our home market. But the big difference between the Spain and the U.S. is that there are no MLSs, so the largest portal is also where you upload the listings, and it’s also pay to list, so an agent is much less likely to list on multiple platforms because it costs them more money. For them we were doing content moderation and categorizing the rooms.
You look at the US, and look at where photos are being uploaded, and that’s how we first learned the acronym, MLS, we had no clue what that was, but there were 700 or so of them. That was five years ago.
Over time, as we became more of a named quantity, but the biggest thing was our more defined product suite.
Did you see this AI wave approaching?
With large language models [OpenAI’s ChatGPT], you’d heard talk of them and seen results behind closed doors, and something we pride ourselves on is reading the different research reports on AI and understanding what’s happening there. But when ChatGPT opened things up and let everyone use it, it did catch us by surprise as to how quickly it was utilized and people started looking all these things you can do.
Ultimately, I think it’s a great thing because before, AI was always three years ago and then ChatGPT comes out and we’re like, ‘Oh, we’re already behind.’ If you look at the big portals — Realtor.com, Zillow, Redfin — within a few months all of them had released some form integration with ChatGPT. It was the tipping point to push things forward which is great for the industry as a whole.
Do you envision a time where AI scans of listing photos is simply part of the process? What could that mean for the space?
That is happening. … There is a growing number of MLSs for which this is part of their listing process. And it’s an opt-out, so as their photos are scanned, whatever is extracted is presented to the agent and they are able to say if it looks good, or if the refrigerator in the photo is not [conveying], they can deselect that. And all that happens in real time.
It would be hard to imagine a world in which this isn’t happening, because of the amount of information and time savings you can get, and we have the data to prove you can get a 30 to 50 percent lift in the amount of data in our MLS by using these services. If you added 100 fields to a listing form and asked agents to do it, well, they wouldn’t have time. But with AI you can continually increase the amount of information you get while only minimally increasing the time agents spend creating that listing.
You have all these things you can do to discover property and they’re used to search and identify different things but those fields aren’t always populated completely, and then things are different in other markets, or items that are subjective, like the style, condition of a property. These are difficult things to get people around the country to standardize. But AI can scan an entire market to create a very granular, very consistent scale for condition, for quality and it allows you to look or things that have never been part of a listing. We can look for the top five markets that have red kitchens in the country. There are a lot of things in that realm we can do but no one ever has because the data hasn’t been there.
What were/are the hardest items for the product to identify in a typical real estate photo?
Everyone thinks AI learns like a human, but it’s not really true, you have a model that knows nothing until you give it a living room or a kitchen, AI only knows what you tell it. We’d run into things early on, if have a living room had a blue square carpet in a room. The AI goes, ‘Well, the only time I’ve seen blue rectangles they’ve been pools.’
Our first client in Canada had snow in some photos, and the AI had never seen snow before, so it’s scrambling trying to figure out what the white stuff is.
While that stuff is kind of funny, we work more in the appraisal space, and as you get into identifying appliances that don’t exist anymore, it becomes hard to train the model.
What if it’s a bad photo, say, too much clutter or bad lighting, can the AI still extract data?
That’s an insightful question. In short, it’s a problem. If a photo is really dark, it may call it a basement, but it’s a bedroom. Well, OK, most photos that are dark are basements, so this feedback lets us know we need to feed it more dark bedrooms so it understands if it also sees a bed and closet it knows it’s a bedroom.
With our quality models it gets tough because you don’t want to say a house is in bad condition because a photo is too dark, but it’s hard to find dark photos of luxury mansions [to help it learn]. We have to at least become more confident that a photo is more one thing than another.
And, [related to agent photos,] we have an internal Slack channel for discussing them.
What are computer vision’s current limitations?
We can detect 600 to 700 different items, but the challenge is you have to continue to train on new things and you have to handle all the corner cases that exist. If we’re auto-populating a listing and we want to see what kind of flooring it has, say concrete floors in the basement and concrete floors in the garage, we don’t want that to be auto-populated, because that’s not what people would expect to see [in a house].
In some photos it may see solar panels, but those panels are on the house next door. So how do we makes sure that doesn’t get populated? The list goes on and on for these, and you have to creative on how you solve those items.
We also have to combine photo data with the data from the MLS, or data from other third party sources, and location data, how do we combine this into an easy-to-consume insight for our user?
What can it learn about exteriors?
We can detect pools, patios, decks, are they covered or not, garage spaces, electric meters, gas meters, exterior quality, and architectural styles, which is one of the most inconsistent things we see nationwide because its called different things in different parts, which is not so helpful when trying to do a study across the country.
But, we did do a study on overcast photos, is it dusk, or if it’s snowy, and studied the impact on price. We found that photos taken in overcast conditions were on the market for an average of 12 days longer. So, those companies doing sky-replacement edits … it’s worth it.
Computer vision can also read video. Does that require more training of the AI, and what do you have planned on that front?
We support video and 360-degree images, and they’re the same underlying technology, but a little bit different in how you determine what you’re looking at. In some parts of a video, it’s looking straight at the room, and that’s great. But you want to make sure the model isn’t returning bad data in those weird-frame moments.
A 5-minute-long video at 60 frames per second comes with a lot more data, and it does it make sense to analyze the data if we also have the video. What purpose does it serve? There are some use cases where it does make sense. We can do it for you, but you might not like the cost. We can process a photo of a property in a second or two. Video takes a lot longer.
How can this technology make a tangible difference in shortening the transaction?
This is important, yes. There is a challenge with buyers and agents, where the buyer says something but means another, and you find that out three months later when they still haven’t found a home. AI can help that.
An agent can ask a client to provide a couple photos of things they like in a home, and the agent can use that to search the market for similar qualities. Let’s say the buyer provided a photo of an industrial living room, or whatever, and those homes exist but are 30 percent above your budget. Or, an agent can tell them where they are, but maybe it’s outside of where they wanted to live. Are these compromises you’re willing to make? Or, you could renovate it after you bought it, or maybe we look for properties that are cheaper and budget that it in.
The other option is, say you’re looking for a modern home on the north side of the city. AI can look at modern homes sold every month, how many are active at a certain time and maybe there’s zero in active inventory right now, so, how important is this component?
Having the data to back up [the search], getting to what compromises the buyer is willing to make sooner, is super valuable for both agent and the client.
What have you learned throughout this process as you’ve tweaked and improved methods?
I feel like every six months there’s a new lesson, but yeah, we’ve learned how difficult it is to do big changes. With an MLS, if they make one change on the UX of a platform, that MLS now has a hundred agents calling asking why they changed it, so trying to navigate how we drive change without creating a burden on the MLSs. We want to solve problems, not create them.
Is there a “holy grail” computer vision application that would be difficult to achieve, but potentially transformative?
If you want to get to that transformative moment, it has to extend beyond computer vision. We started as a photo company. We tell you what’s in a photo. Then we were able to look at all the photos at one time, and that was a big thing, because then we can say things about property, not just a particular image. Now, imagine that insight spread across a market, updated every day.
Now imagine you’re a selling agent, and you learn of multiple buyers looking for something that doesn’t exist, but you know a homeowner who has one, you could go to them and prove that there’s unusual demand and short supply for homes like yours, are you willing to sell? There’s a subset of people who would be interested in that, and that could lead to greater liquidity across the market, all enabled by the data that’s already there.
The best thing data can do for you is confirm what you’re saying, and it can take the mental load off the agent to provide answers.